Writing | Zuo Chao Qian Jiaming
In March 2016, DeepMind, a Google-owned artificial intelligence (AI) company, defeated Go world champion Lee Sedol 4:1 with its AlphaGo artificial intelligence system, triggering a new wave of artificial intelligence – deep learning technology. Since then, people have witnessed the rapid rise and wide application of deep learning technology – it has solved many problems and challenges in computer vision, computational imaging, and computer-aided diagnostics with unprecedented performance. At the same time, Google, Facebook, Microsoft, Apple, and Amazon, the five tech giants without exception, are investing more and more resources to seize the artificial intelligence market, and even transforming into artificial intelligence-driven companies as a whole. They have begun to "ignite" the "art" of data mining and developed easy-to-use open-source deep learning frameworks. These deep learning frameworks enable us to use pre-built and optimized component sets to build complex, large-scale deep learning models in a clearer, concise, and user-friendly way without having to delve into the details of the underlying algorithms. Domestic "BAT" also regards deep learning technology as a key strategic direction, and actively deploys the field of artificial intelligence with its own advantages. Deep learning has rapidly left the halls of academia and is beginning to reshape industry.
Optical metrology, on the other hand, is a type of measurement science and technology that uses optical signals as the standard/information carrier. It is an ancient discipline, because the development of physics has been driven by optical metrology from the very beginning. But in turn, optical metrology has also undergone major changes with the invention of lasers, charge-coupled devices (CCDs), and computers. It has now developed into a broad interdisciplinary field and is closely related to disciplines such as photometry, optical engineering, computer vision, and computational imaging. Given the great success of deep learning in these related fields, researchers in optical metrology cannot suppress their curiosity and have begun to actively participate in this rapidly developing and emerging field. Unlike traditional methods based on "physics a priori", "data-driven" deep learning technology offers new possibilities for solving many challenging problems in the field of optical metrology, and shows great application potential.
In this context, in March 202,Nanjing University of Science and TechnologywithNanyang Technological University, SingaporeThe research team published in the top international optical journal "Lighting: Science & Applications"A joint statement entitled"Deep learning in optical metrology: a review"The first author of the review article is Nanjing University of Science and TechnologyZuo ChaoProfessor, PhD student at Nanjing University of Science and TechnologyQian JiamingCo-first author, Nanjing University of Science and TechnologyZuo Chao,Chen QianProfessor, Nanyang Technological University, SingaporeChandler SpearProfessor is the co-corresponding author of the paper, and Nanjing University of Science and Technology is the first unit of the paper.
This paper systematically summarizes the classical techniques and image processing algorithms in optical metrology, briefly describes the development history, network structure and technical advantages of deep learning, and comprehensively reviews its specific applications in various optical metrology tasks (such as fringe denoising, phase demodulation and phase unwrapping). By comparing the similarities and differences in principle and thought between deep learning methods and traditional image processing algorithms, the unique advantages of deep learning in solving "problem reconstruction" and "actual performance" in various optical metrology tasks are demonstrated. Finally, the paper points out the challenges faced by deep learning technology in the field of optical metrology, and looks forward to its potential future development direction.
Traditional optical metrology
Image generation model and image processing algorithm
Optical metrology technology cleverly uses the basic properties of light (such as amplitude, phase, wavelength, direction, frequency, speed, polarization and coherence, etc.) as the information carrier of the measured object to realize the acquisition of various characteristic data of the measured object (such as distance, displacement, size, morphology, roughness, strain and stress, etc.). Optical metrology has been increasingly widely used in CAD /CAE, reverse engineering, online detection, quality control, medical diagnosis, cultural relics protection, human-interaction machine and other fields due to its advantages of non-contact, high speed, high sensitivity, high resolution and high accuracy.In optical metrology, the most common information carriers are "streaks" and "speckles."For example, the images processed by most interferometry methods (classical interference, photoelasticity, digital speckle, digital holography, etc.) are interference fringes formed by the coherent superposition of object light and reference light, and the measured physical quantity is modulated in the phase information of the interference fringes. In addition, the fringe pattern can also be generated in a non-interferometric way, such as fringe projection profilometry (FPP) directly projecting the fringe pattern of structured light to the surface of the measured object to measure the three-dimensional surface shape of the object. In digital image correlation (DIC), the captured image is the speckle pattern before and after the deformation of the sample surface, from which the total field displacement and deformation distribution of the measured object can be obtained. Combining DIC with stereo vision or photogrammetry, the depth information of the measured scene can also be obtained based on multi-view speckle images. Figure 1 summarizes the image generation process of these techniques and their corresponding mathematical models.
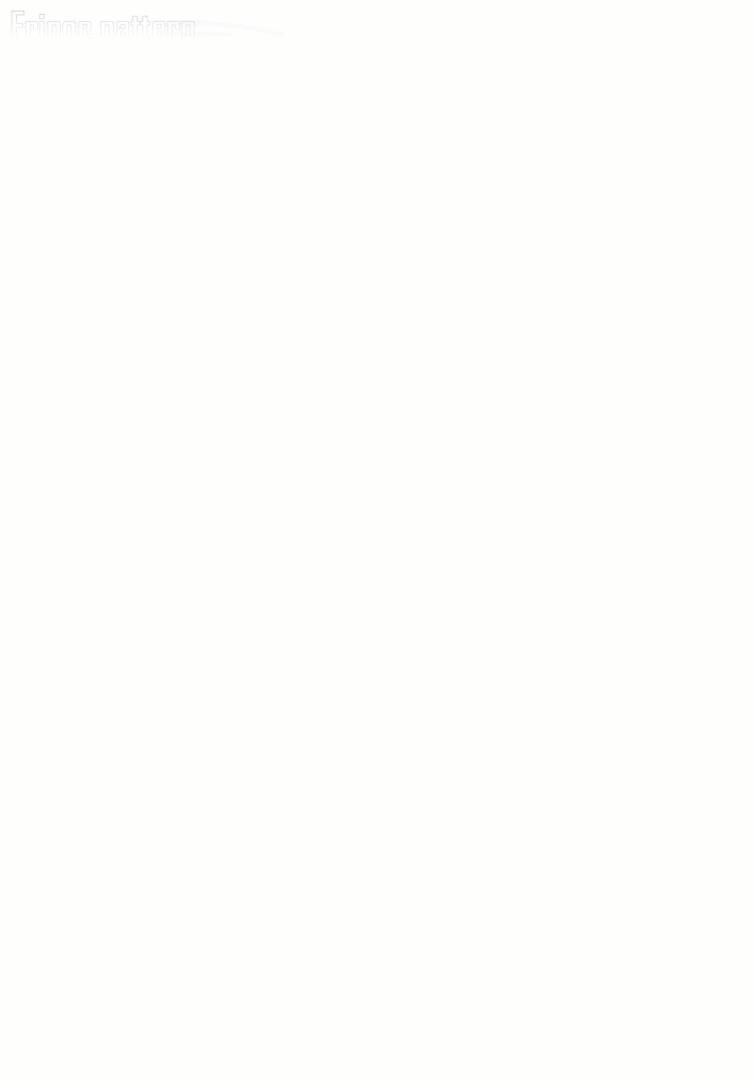
Figure 1 The image generation process and corresponding mathematical model in traditional optical metrology technology
Traditional optical metrology is inseparable from image processing technologyImage processing of fringe/speckleIt can be understood as a process of inverting the required physical quantity to be measured from the captured original intensity image. Usually, this process is not "Instead, it consists of three logically hierarchical image processing steps – pre-processing, analysis, and post-processing.Each step involves a series of image processing algorithms, which are layered on top of each other to form a "pipeline" structure [Figure 2], where each algorithm corresponds to a "map"Operation, which converts the matrix input of an image/similar image into the output of the corresponding dimension (or resampling)."
(1) PretreatmentImage preprocessing improves image quality by suppressing or minimizing unnecessary interference signals (such as noise, aliasing, distortion, etc.). Representative image preprocessing algorithms in optical metrology include image denoising, image enhancement, color channel separation, and image registration and correction.
(2) Analysis: Image analysis is the core step of image processing algorithms, which is used to extract important information carriers related to the physical quantities to be measured from the input image. In phase measurement technology, the main task of image analysis is to reconstruct phase information from fringe images. The basic algorithms include phase demodulation and phase unfolding. For stereo matching technology, image analysis refers to determining the displacement vector between points corresponding to the speckle image (the speckle pattern before and after the deformation of the sample surface/the multi-view speckle image), which generally includes two steps of subset matching and sub-pixel optimization.
(3) Post-processing:The purpose of image post-processing is to further optimize the measured phase data or speckle displacement fields and eventually convert them into physical quantities to be measured. Common post-processing algorithms in optical metrology include noise removal, error compensation, digital refocus, and parameter conversion. Figure 3 provides an overview of the image processing hierarchy of optical metrology and various image processing algorithms distributed in different layers.
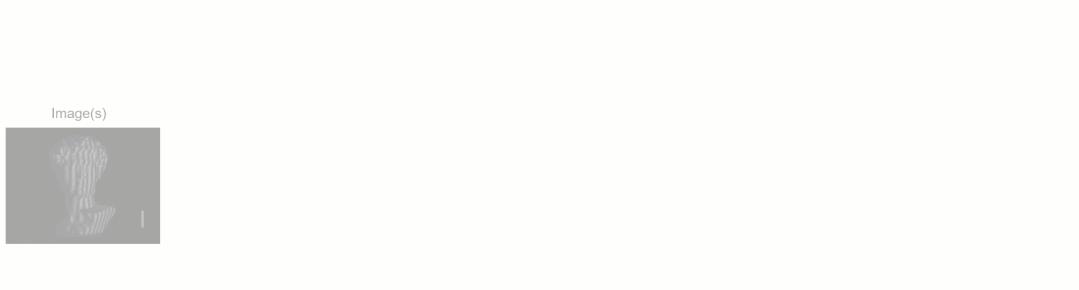
A typical image processing process for optical metrology (e.g. fringe projection profiling) can be divided into three main steps: preprocessing (e.g. denoising, image enhancement), analysis (e.g. phase demodulation, phase unwrapping), and post-processing (e.g. phase-depth mapping).
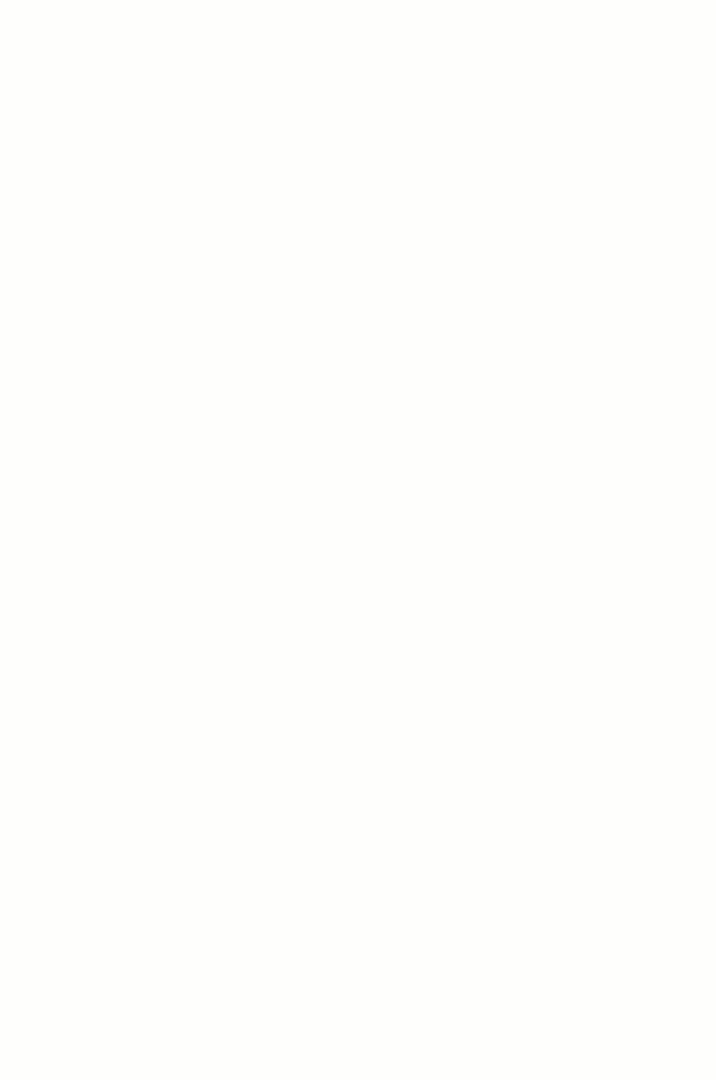
Figure 3 Overview of the optical metrology image processing hierarchy and various image processing algorithms distributed in different layers
Deep learning technology
Principle, development and convolutional neural networks
Deep learning is an important branch in the field of machine learning. It builds neural structures that simulate the information processing of the human brainArtificial neural networks (ANN), enabling machines to perform bottom-up feature extraction from large amounts of historical data, thus enabling intelligent decision-making on future/unknown samples. ANN originated from a simplified mathematical model of biological nerve cells established by McCulloch and Pitts in 1943 2 ?? [Fig. 4a]. In 1958, Rosenblatt et al 2 ??, inspired by the biological nerve cell model, first proposed a machine that could simulate human perceptual abilities – a single-layer perceptron. As shown in Fig. 4b, a single-layer perceptron consists of a single nerve cell. The nerve cell maps the input to the output through a non-linear activation function with bias (b) and weight (w) as parameters. The proposal of perceptrons has aroused the interest of a large number of researchers in ANNs, which is a milestone in the development of neural networks. However, the limitation that single-layer perceptrons can only handle linear classification problems has caused the development of neural networks to stagnate for nearly 20 years. In the 1980s, the proposal of backpropagation (BP) algorithm made it possible to train multi-layer neural networks efficiently. It continuously adjusts the weights between nerve cells based on the chain rule to reduce the output error of multi-layer networks, effectively solving the problem of nonlinear classification and learning, triggering a boom in "shallow learning" 2 2. In 1989, LeCun et al. 2 3 proposed the idea of convolutional neural networks (CNNs) inspired by the structure of mammalian visual cortex, which laid the foundation for deep learning for modern computer vision and image processing. Subsequently, as the number of layers of neural networks increased, the problem of layer disappearance/explosion of BP algorithm became increasingly prominent, which caused the development of ANN to stagnate in the mid-1990s. In 2006, Hinton et al. proposed a deep belief network (DBN) training method to deal with the problem of layer disappearance; at the same time, with the development of computer hardware performance, GPU acceleration technology, and the emergence of a large number of labeled datasets, neural networks entered the third development climax, from the "shallow learning" stage to the "deep learning" stage. In 2012, AlexNet based on CNN architecture won the ImageNet image recognition competition in one fell swoop, making CNN one of the mainstream frameworks for deep learning after more than 20 years of silence. At the same time, some new deep learning network architectures and training methods (such as ReLU 2 and Dropout 2)It was proposed to further solve the problem of layer disappearance, which promoted the explosive growth of deep learning. In 2016, AlphaGo, an artificial intelligence system developed by Google’s AI company DeepMind, defeated Lee Sedol, the world champion of Go, which aroused widespread attention to deep learning technology among all mankind 2. Figure 4 shows the development process of artificial neural networks and deep learning technologies and the structural diagram of typical neural networks.
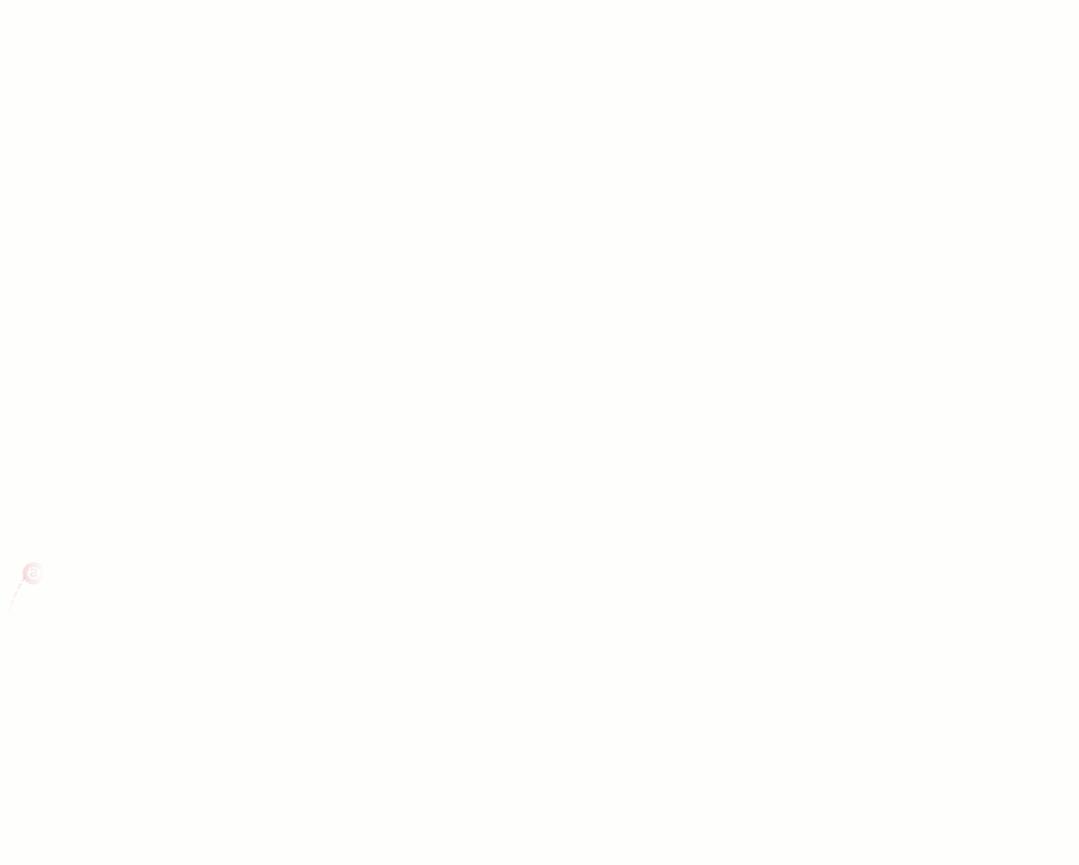
Figure 4 The development process of deep learning and artificial neural networks and the structural diagram of typical neural networks
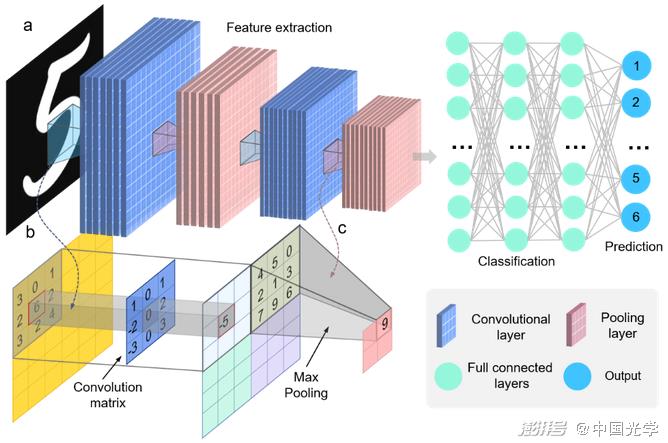
Figure 5 Typical CNN structure for image classification tasks
A) A typical CNN consists of an input layer, a convolutional layer, a fully connected layer, and an output layer b) a convolutional operation c) a pooling operation
The single-layer perceptron described above is the simplest ANN structure and consists of only a single nerve cell [Fig. 4b]. Deep neural networks (DNNs) are formed by connecting multiple layers of nerve cells to each other, with nerve cells between adjacent layers typically stacked in a fully connected form [Fig. 4g]. During network training, the nerve cell multiplies the corresponding input by a weight coefficient and adds it to the bias value, outputting it to the next layer through a non-linear activation function, while network losses are computed and backpropagated to update network parameters. Unlike conventional fully connected layers, CNNs use convolutional layers to perform feature extraction on the input data 2 [Fig. 5a]. In each layer, the input image is convoluted with a set of convolutional filters and added biases to generate a new output image [Fig. 5b]. Pooling layers in CNNs take advantage of the local correlation principle of the image to subsample the image, reducing the amount of data processing while preserving useful information [Fig. 5c]. These features make CNNs widely used in tasks of computer vision, such as object detection and motion tracking. Traditional CNN architectures are mostly oriented towards "classification" tasks, discarding spatial information at the output and producing an output in the form of a "vector". However, for image processing tasks in optical metrology techniques, neural networks must be able to produce an output with the same (or even higher) full resolution as the input. For this purpose, a fully convolutional network architecture without a fully connected layer should be used. Such a network architecture accepts input of any size, is trained with regression loss, and produces pixel-level matrix output. Networks with such characteristics are called "fully convolutional network architectures" CNNs, and their network architectures mainly include the following three categories:
(1) SRCNN:Dong et al. 3 2 skip the pooling layer in the traditional CNN structure and use a simple stacking of several convolutional layers to preserve the input dimension at the output [Fig. 6a]. SRCNN constructed using this idea has become one of the mainstream network frameworks for image super-resolution tasks.
(2) FCN:A fully convolutional network (FCN) is a network framework for semantic segmentation tasks proposed by Long et al. As shown in Figure 6b, FCN uses the convolutional layer of a traditional CNN [Fig. 5] as the network coding module and replaces the fully connected layer with a deconvolutional layer as the decoding module. The deconvolutional layer is able to upsample the feature map of the last convolutional layer so that it recovers to an output of the same size as the input image. In addition, FCN combines coarse high-level features with detailed low-level features through a skip structure, allowing the network to better recover detailed information while preserving pixel-level output.
(3) U-Net:Ronneberger et al. made improvements to FCN and proposed U-Net network 3. As shown in Figure 6c, the basic structure of U-Net includes a compressed path and an extended path. The compressed path acts as the encoder of the network, using four convolutional blocks (each convolutional block is composed of three convolutional layers and a pooling layer) to downsample the input image and obtain the compressed feature representation; the extended path acts as the network decoder using the upsampling method of transposed convolution to output the prediction result of the same size as the input. U-Net uses jump connection to perform feature fusion on the compressed path and the extended path, so that the network can freely choose between shallow features and deep features, which is more advantageous for semantic segmentation tasks.
The above-mentioned fully convolutional network structure CNN can convert input images of any size into pixel-level matrix output, which is completely consistent with the input and output characteristics of the "mapping" operation corresponding to the image processing algorithm in the optical metrology task, so it can be very convenient to "deep learning replacement" for traditional image processing tasks, which laid the foundation for the rapid rise of deep learning in the field of optical metrology.
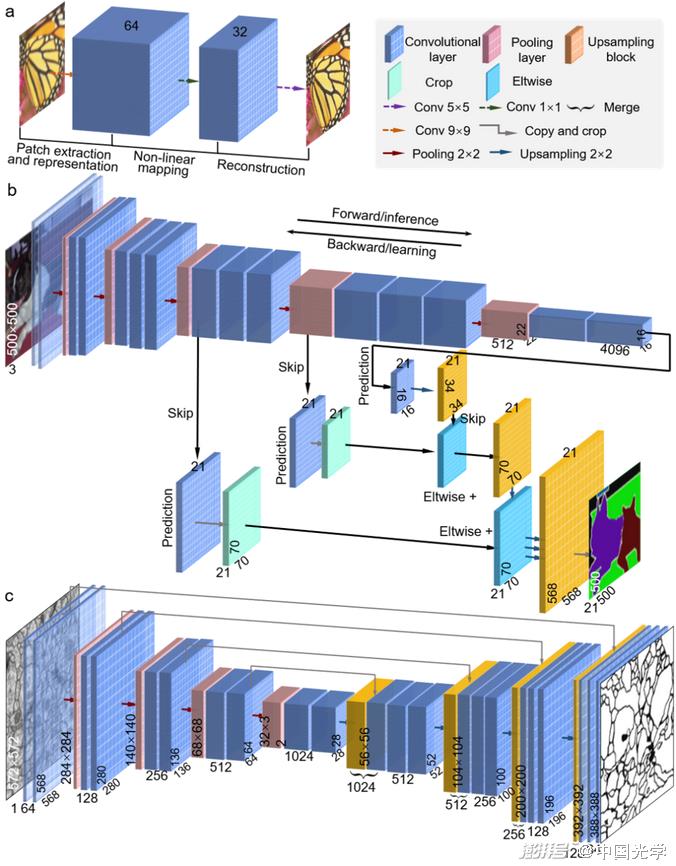
Fig.6 Three representative fully convolutional network architectures of CNNs capable of generating pixel-level image output for image processing tasks
A) SRCNN b) FCN c) U-Net.
Optical metrology in deep learning
Changes in thinking and methodology
In optical metrology, the mapping between the original fringe/speckle image and the measured physical quantity can be described as a combination of forward physical model and measurement noise from parameter space to image space, which can explain the generation process of almost all original images in optical metrology. However, extracting the physical quantity to be measured from the original image is a typical "inverse problem". Solving such inverse problems faces many challenges, such as: unknown or imprecise forward physical model, error accumulation and local optimal solution, and pathology of inverse problems. In the field of computer vision and computational imaging, the classic method for solving inverse problems is to define the solution space by introducing the prior of the measured object as a regularization means to make it well-conditioned [Figure 7]. In the field of optical metrology, the idea of solving the inverse problem is quite different. The fundamental reason is that optical metrology is usually carried out in a "highly controllable" environment, so it is more inclined to "actively adjust" the image acquisition process through a series of "active strategies", such as lighting modulation, object regulation, multiple exposures, etc., which can reshape the original "sick inverse problem" into a "well-conditioned and sufficiently stable regression problem". For example, demodulating the absolute phase from a single fringe image: the inverse problem is ill-conditioned due to the lack of sufficient information in the forward physical model to solve the corresponding inverse problem uniquely and stably. For researchers in optical metrology, the solution to this problem is very simple: we can make multiple measurements, and by acquiring additional multi-frequency phase-shifted fringe images, the absolute phase acquisition problem evolves into a good-state regression problem. We can easily recover the absolute phase information of the measured object from these fringe images by multi-step phase-shifting and time-phase unwrapping [Figure 8].
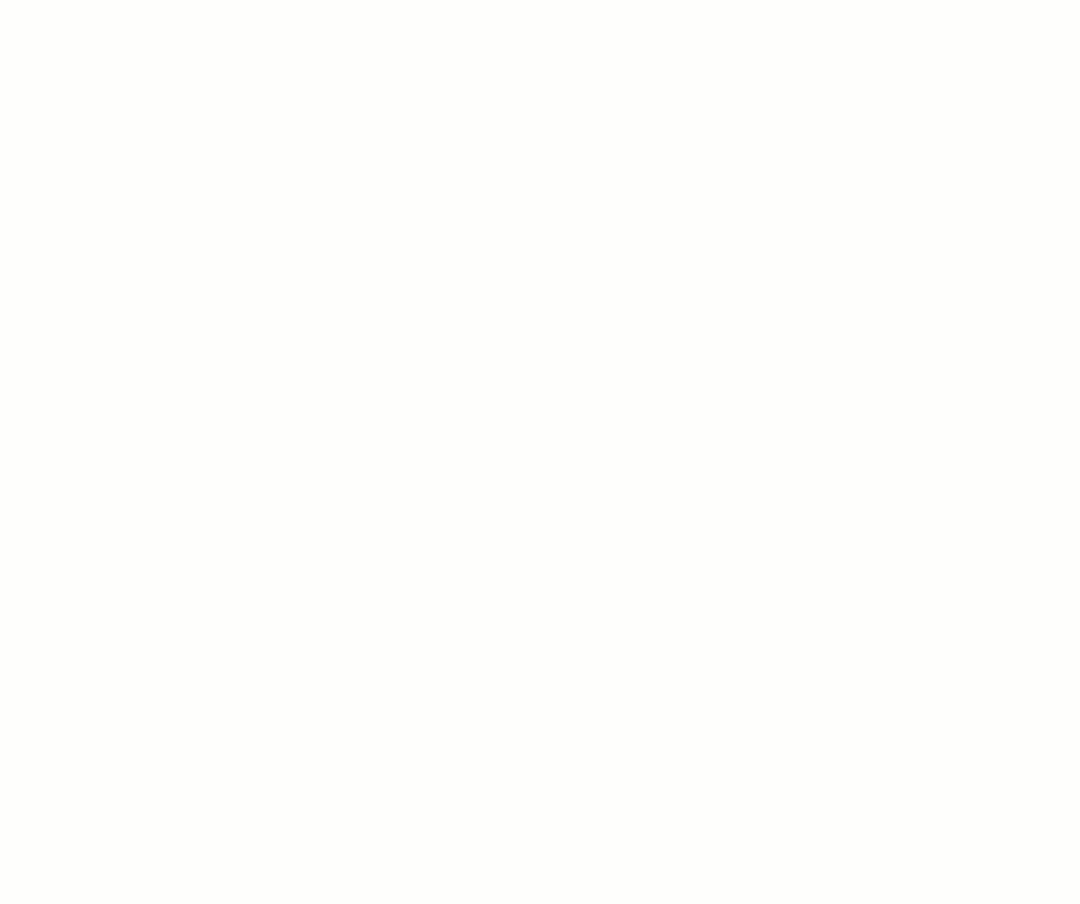
Figure 7 In computer vision (e.g. image deblurring), the inverse problem is ill-conditioned because the forward physical model mapped from the parameter space to the image space is not ideal. A typical solution is to reformulate the original ill-conditioned problem as a well-conditioned optimization problem by adding some prior assumptions (smoothing) that aid regularization
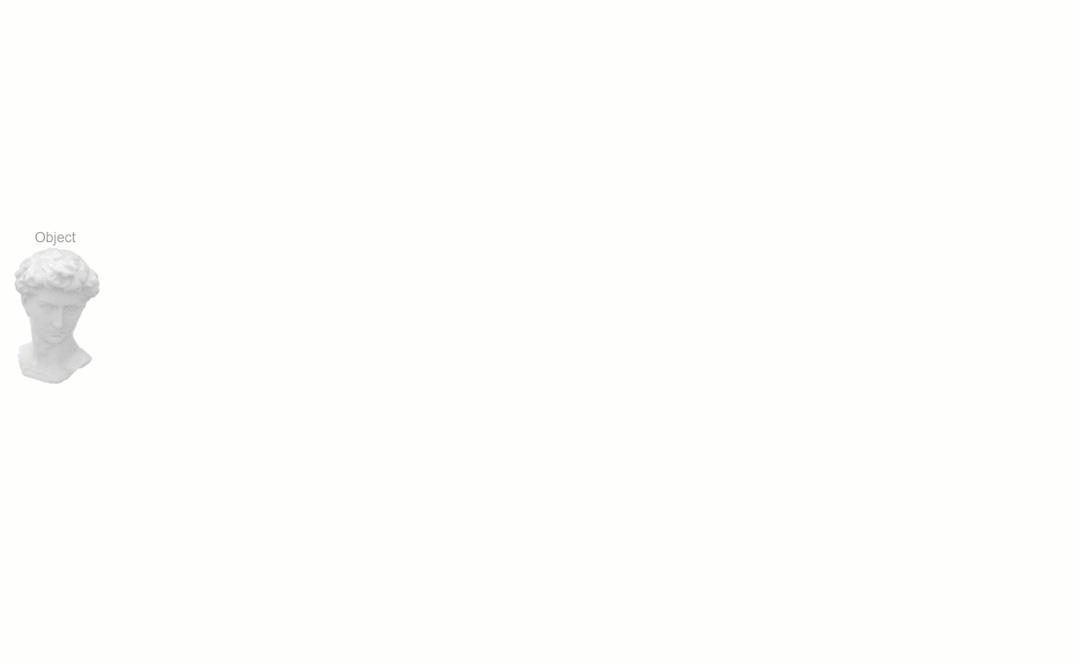
Fig. 8 Optical metrology transforms a ill-conditioned inverse problem into a well-conditioned regression problem by actively controlling the image acquisition process. For example, in fringe projection profilometry, by acquiring additional phase-shifted fringe images of different frequencies, the absolute phase can be easily obtained by multi-frequency phase-shift method and temporal phase expansion method
However, when we step out of the laboratory and into the complex environment of the real world, the situation can be very different. The above active strategies often impose strict restrictions on the measurement conditions and the object being measured, such as:Stable measurement system, minimal environmental disturbance, static rigid objects, etcHowever, for many challenging applications, such as harsh operating environments and fast-moving objects, the above active strategy may become a "Luxury"Even impractical requirements. In this case, traditional optical metrology methods will face serious physical and technical limitations, such as limited data volume and uncertainty of forward models.How to extract high-precision absolute (unambiguous) phase information from minimal (preferably single-frame) fringe patterns remains one of the most challenging problems in optical metrology today.Therefore, we look forward to innovations and breakthroughs in the principles and methods of optical metrology, which are of great significance for its future development.
As a "data-driven" technology that has emerged in recent years, deep learning has received more and more attention in the field of optical metrology and has achieved fruitful results in recent years. Different from traditional physical model-driven methods,The deep learning method creates a set of training datasets composed of real target parameters and corresponding original measurement data, establishes their mapping relationships using ANN, and learns network parameters from the training dataset to solve the inverse problem in optical metrology[Figure 9]. Compared to traditional optical metrology techniques, deep learning moves active strategies from the actual measurement phase to the network training phase, gaining three unprecedented advantages:
1) From "model-driven" to "data-driven"Deep learning overturns the traditional "physical model-driven" approach and opens up a new paradigm based on "data-driven". Reconstruction algorithms (inverse mappings) can learn from experimental data without prior knowledge of physical models. If the training dataset is collected based on active strategies in a real experimental environment (including measurement systems, sample types, measurement environments, etc.), and the amount of data is sufficient (diversity), then the trained model should be able to reflect the real situation more accurately and comprehensively, so it usually produces more accurate reconstruction results than traditional physical model-based methods.
(2) From "divide and conquer" to "end-to-end learning":Deep learning allows for "end-to-end" learning of structures in which neural networks can learn a direct mapping relationship between raw image data and the desired sample parameters in one step, as shown in Figure 10, compared to traditional optical metrology methods of independently solving sequences of tasks. The "end-to-end" learning method has the advantage of synergy compared to "step-by-step divide-and-conquer" schemes: it is able to share information (features) between parts of the network performing different tasks, contributing to better overall performance compared to solving each task independently.
(3) From "solving linear inverse problems" to "directly learning pseudo-inverse maps": Deep learning uses complex neural networks and nonlinear activation functions to extract high-dimensional features of sample data, and directly learns a nonlinear pseudo-inverse mapping model ("reconstruction algorithm") that can fully describe the entire measurement process (from the original image to the physical quantity to be measured). For regularization functions or specified priors than traditional methods, the prior information learned by deep learning is statistically tailored to real experimental data, which in principle provides stronger and more reasonable regularization for solving inverse problems. Therefore, it bypasses the obstacles of solving nonlinear ill-conditioned inverse problems and can directly establish the pseudo-inverse mapping relationship between the input and the desired output.
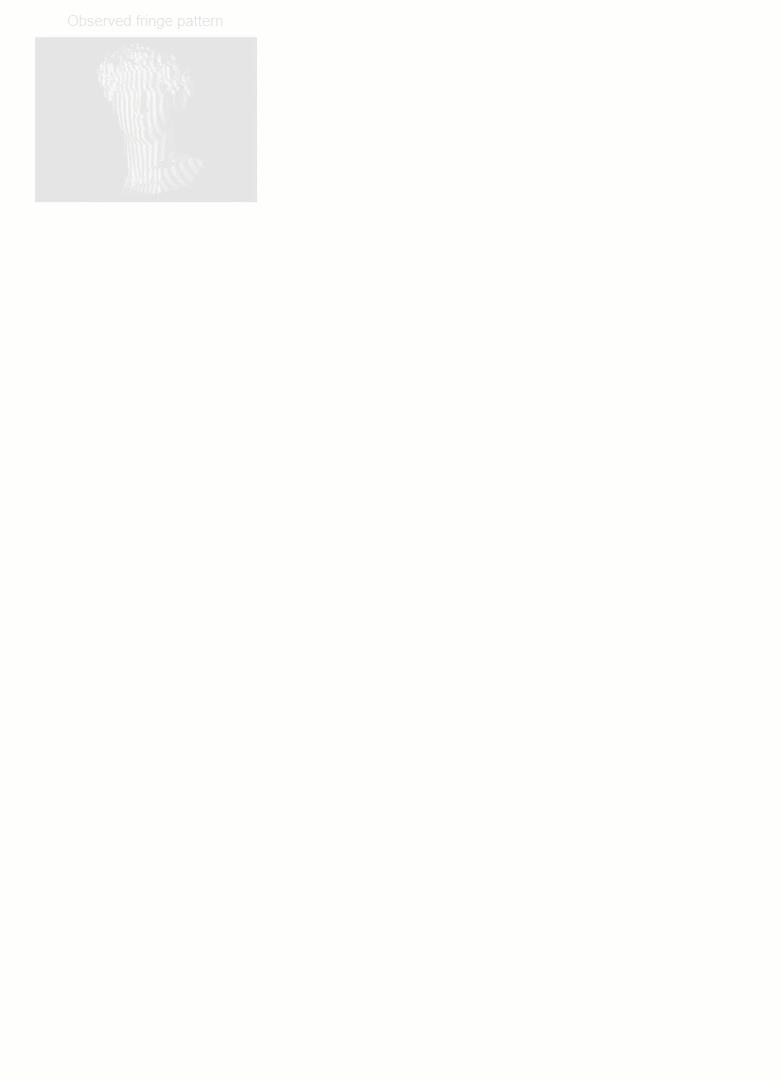
Fig. 9 Optical metrology based on deep learning
A) In deep learning-based optical metrology, the mapping of image space to parameter space is learned from a dataset by building a deep neural network b) The process of obtaining a training dataset through experimentation or simulation.
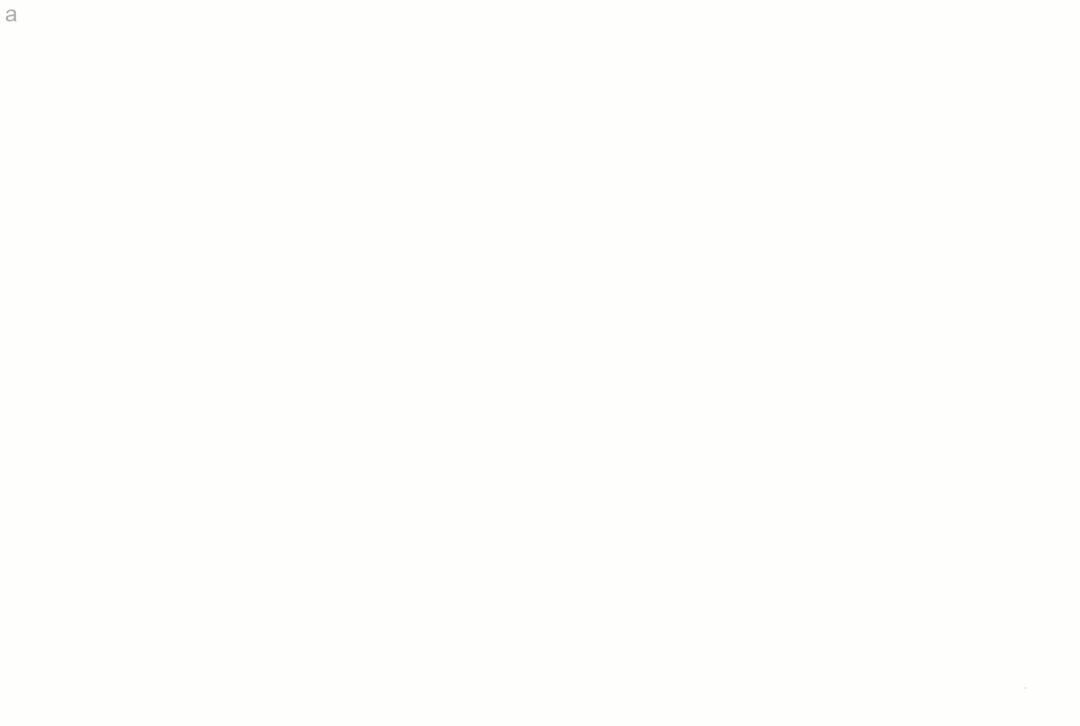
Figure 10 Comparison of deep learning and traditional algorithms in the field of fringe projection
A) The basic principle of fringe projection profiling is 3D reconstruction based on optical triangulation (left). Its steps generally include fringe projection, phase recovery, phase unwrapping, and phase-height mapping b) Deep learning-based fringe projection profiling is driven by a large amount of training data, and the trained network model can directly predict the encoded depth information from a single frame of fringes
Application of deep learning in optical metrology
A complete revolution in image processing algorithms
Due to the above advantages, deep learning has received more and more attention in optical metrology, bringing a subversive change to the concept of optical metrology technology. Deep learning abandons the strict reliance on traditional "forward physical models" and "reverse reconstruction algorithms", and reshapes the basic tasks of digital image processing in almost all optical metrology technologies in a "sample data-driven" way. Breaking the functional/performance boundaries of traditional optical metrology technologies, mining more essential information of scenes from very little raw image data, significantly improving information acquisition capabilities, and opening a new door for optical metrology technology.Figure 11 reviews typical research efforts using deep learning techniques in the field of optical metrology. Below are specific application cases of deep learning in optical metrology according to the image processing level of traditional optical metrology techniques.
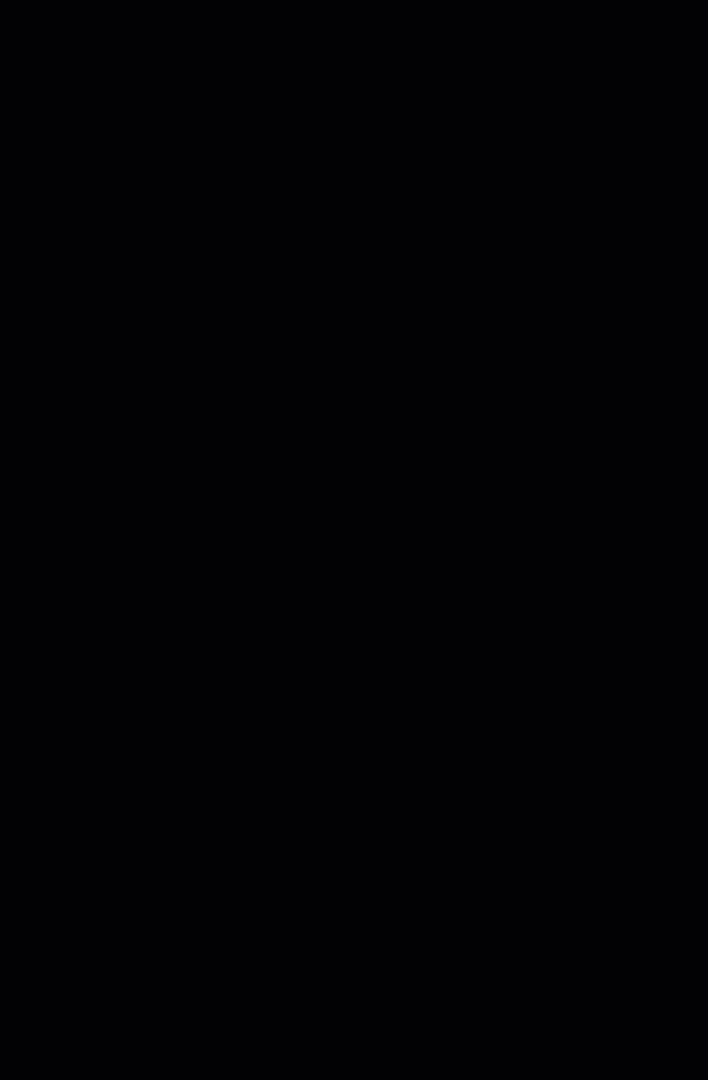
Figure 11 Deep learning in optical metrology: Since deep learning has brought significant conceptual changes to optical metrology, the implementation of almost all tasks in optical metrology has been revolutionized by deep learning
(1) Image preprocessing:Early work on applying deep learning to optical metrology focused on image preprocessing tasks such as image denoising and image enhancement. Yan et al. constructed a CNN composed of 20 convolutional layers to achieve fringe image denoising [Fig. 12a]. Since noise-free ideal fringe images are difficult to obtain experimentally, they simulated a large number of fringe images with Gaussian noise added (network input) and corresponding noise-free data (true value) as training datasets for neural networks. Figures 12d-12e show the denoising results of traditional denoising methods – windowed Fourier transform (WFT 3) and deep learning methods. It can be seen from the results that the deep learning-based method overcomes the edge artifacts of traditional WFT and exhibits better denoising performance. Shi et al. proposed a deep learning-based method for fringe information enhancement [Fig. 13a]. They used the fringe images captured in real scenes and the corresponding quality-enhanced images (acquired by subtracting two fringe images with a phase shift of π) as a dataset to train neural networks to achieve a direct mapping between the fringe images to the quality-enhanced fringe information. Fig. 13b-Fig. 13d shows the results of the 3D reconstruction of the moving hand by the traditional Fourier transform (FT) 3 and deep learning methods. From this, it can be seen that the deep learning method is significantly better than the traditional method in imaging quality.
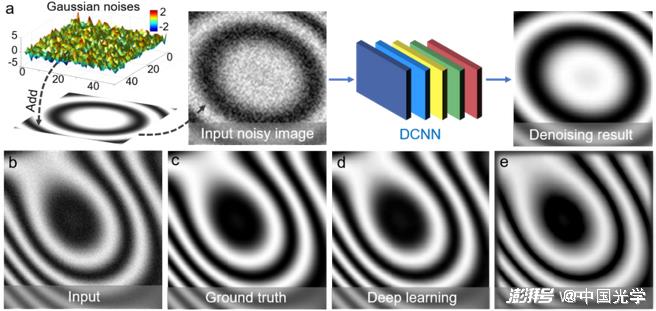
Figure 12 The denoising method of fringe image based on deep learning and the denoising results of different methods.
A) The process of fringe denoising using depth learning: the fringe image with noise is used as the input of neural networks to directly predict the denoised image b) the input noise image c) the true phase distribution d) the denoising result of deep learning e) the denoising result of WFT 3
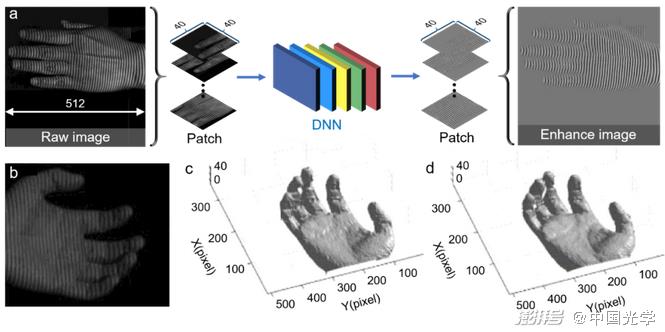
Fig. 13 Fringe information enhancement method based on deep learning and 3D reconstruction results under different methods.
A) using depth learning for fringe information addition process: the original fringe image and the acquired quality enhancement image are used to train DNN to learn the mapping between the input fringe image and the output quality enhancement fringe information b) input fringe image c) conventional FT method 38 3D reconstruction results d) 3D reconstruction results of deep learning method
(2) Image analysis:Image analysis is the most core image processing link in optical metrology technology, so most deep learning techniques applied to optical metrology are for processing tasks related to image analysis. For phase measurement technology, deep learning has been widely explored in phase demodulation and phase unwrapping. Zuo et al. applied deep learning technology to fringe analysis for the first time, and effectively improved the three-dimensional measurement accuracy of FPP. The idea of this method is to use only one fringe image as input, and use CNN to simulate the phase demodulation process of the traditional phase shift method. As shown in Figure 14a, two convolutional neural networks (CNN1 and CNN 2) are constructed, where CNN1 is responsible for processing the fringe image from the input (IExtract background information (ACNN 2 then uses the extracted background image and the sinusoidal portion of the desired phase of the original input image generation.M) and the cosine part (D); Finally, the output sine-cosine result is substituted into the arctangent function to calculate the final phase distribution. Compared with the traditional single-frame phase demodulation methods (FT 3 and WFT 3), the deep learning-based method can extract phase information more accurately, especially for the surface of objects with rich details, and the phase accuracy can be improved by more than 50%. Only one input fringe image is used, but the overall measurement effect is close to the 12-step phase shift method [Fig. 14b]. This technology has been successfully applied to high-speed 3D imaging, achieving high-precision 3D surface shape measurement up to 20000Hz [Fig. 14c]. Zuo et al. further generalized deep learning from phase demodulation to phase unwrapping, and proposed a deep learning-based geometric phase unwrapping method for single-frame 3D topography measurement. As shown in Figure 15a, the stereo fringe image pairs and reference plane information captured under the multi-view geometric system are fed into the CNN to determine the fringe order. Figures 15b-15e show the 3D reconstruction results obtained by the traditional geometric phase unwrapping method and the deep learning method. These results show that the deep learning-based method can achieve phase unwrapping of dense fringe images in a larger measurement volume and more robustly under the premise of projecting only a single frame of fringe images.
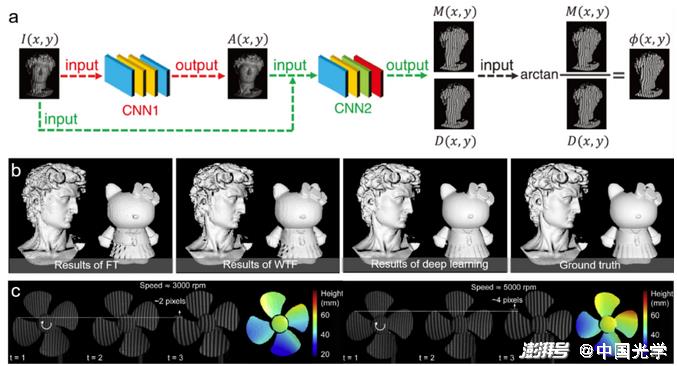
Fig. 14 Fringe analysis method based on deep learning and three-dimensional reconstruction results under different methods 3 a) Fringe analysis method flow based on deep learning: First, the background image A is predicted from the single frame fringe image I by CNN1; then CNN2 is used to realize the fringe pattern I, The mapping between the background image A and the sinusoidal part M and the cosine part D that generate the desired phase; finally, the phase information can be wrapped with high accuracy through the tangent function b) Comparison of three-dimensional reconstruction of different phase demodulation methods (FT 3, WFT 3, deep learning-based method and 12-step phase shift method 3 3) c) Deep reconstruction results of a high-speed rotating table fan using depth learning method
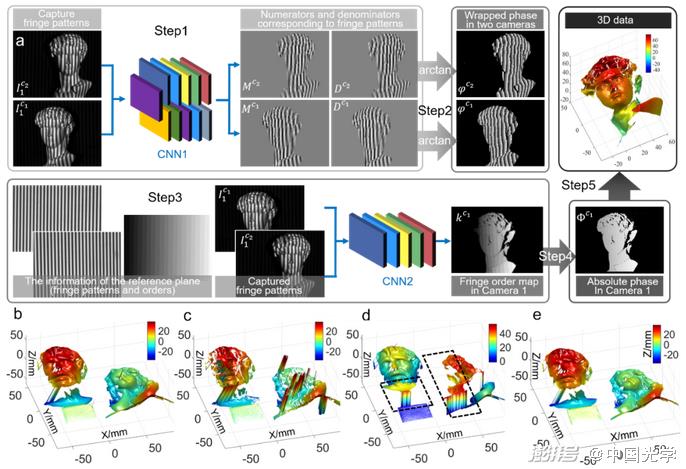
Fig. 15 Geometric phase unwrapping method based on deep learning and 3D reconstruction results under different methods < unk > a) Flow of geometric phase unwrapping method assisted by deep learning: CNN1 predicts the wrapping phase information from the stereo fringe image pair, CNN2 predicts the fringe order from the stereo fringe image pair and reference information. The absolute phase can be recovered by the predicted wrapping phase and fringe order, and then 3D reconstruction is performed b) 3D reconstruction results obtained by combining phase shift method, three-camera geometric phase expansion technique, and adaptive depth constraint method, c) 3D reconstruction results obtained by combining phase shift method, two-camera geometric phase expansion technique, d) 3D reconstruction results obtained by geometric constraint method based on reference surface, e) 3D reconstruction results obtained by deep learning method
Deep learning is also widely used for stereo matching and achieves better performance than traditional subset matching and sub-pixel optimization methods. Zbontar and LeCun ?? propose a deep learning method for stereo image disparity estimation [Fig. 16]. They constructed a Siamese-type CNN to solve the matching cost calculation problem by learning similarity metrics from two image blocks. The output of the CNN is used to initialize the stereo matching cost, and then to achieve disparity map estimation by refining the initial cost through cross-based cost aggregation and semi-global matching. Fig. 16d-Fig. 16h are disparity images obtained by traditional Census transformation and deep learning methods. From this, it can be seen that the deep learning-based method achieves lower error rates and better prediction results. Pang et al. propose a cascaded CNN architecture for sub-pixel matching. As shown in Figure 17a, the initial disparity estimation is first predicted from the input stereo image pair by DispFulNet with upsampling module, and then the multi-scale residual signal is generated by the hourglass-structured DispResNet, which synthesizes the output of the two networks and finally obtains the disparity map with sub-pixel accuracy. Figures 17d-17g show the disparity map and error distribution predicted by DispfulNet and DispResNet. It can be seen from the experimental results that the quality of the disparity map has been significantly improved after the optimization of DispResNet in the second stage.
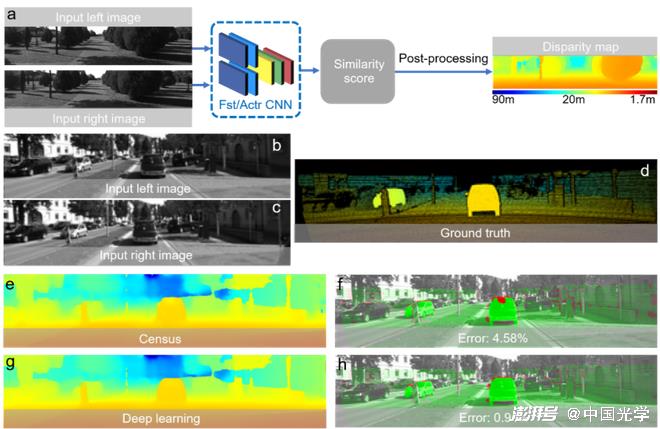
Figure 16 The disparity estimation results of the subset matching method based on deep learning and the disparity estimation results of different methods ?? a) The algorithm flow of disparity map estimation using depth learning: Siamese CNN is constructed to learn similarity metrics from two image blocks to solve the matching cost calculation problem, and finally realizes the disparity estimation through a series of post-processing b-c) The input stereo image d) true value e, g) Census and the disparity estimation results obtained by CNN
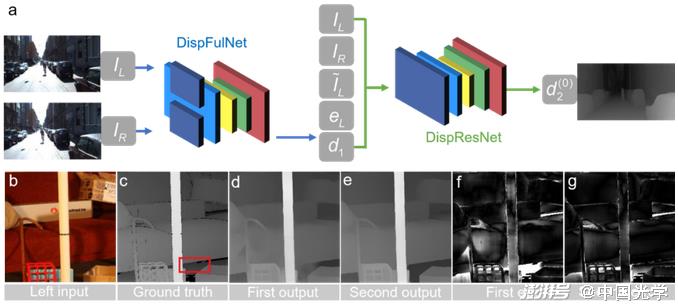
Figure 17 a) Sub-pixel matching method based on deep learning: First, the initial disparity estimation is predicted from the input stereo image pair through DispFulNet, and then the multi-scale residual signal is generated through the hourglass structure DispResNet, and the final output of the two networks is obtained. The disparity map with sub-pixel accuracy b) the left viewing angle of the input stereo image c) true value d-g) the disparity map and error distribution predicted by DispfulNet and DispResNet
(3) Post-processing: Deep learning also plays an important role in the post-processing phase of optical metrology (phase denoising, error compensation, digital refocus, phase-height mapping, etc.). As shown in Figure 18a, Montresor et al. input the sine and cosine components of the noise phase image into the CNN to predict the noise-removed high-quality phase image, and the predicted phase is fed back to the CNN for iterative refining to achieve better denoising effect. Figures 18b-18e show the phase denoising results of the traditional WFT 3 method and the deep learning method. Experimental results show that the CNN can achieve lower denoising performance than the WFT peak-valley phase error.
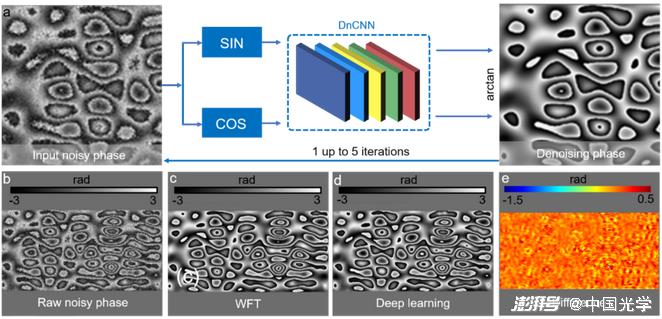
Figure 18 Phase denoising method based on deep learning and phase denoising results of different methods a) The process of phase denoising using depth learning: the sine and cosine components of the noise phase image are input to the CNN to predict the high-quality phase image with noise removal, and the predicted phase is fed back to the CNN again for iterative refining to achieve better denoising effect b) input noise phase image c) denoising result of WTF 3 d) denoising result of deep learning e) Comparison of WTF and deep learning method denoising results
Li et al. proposed a phase-height mapping method for fringe projection profilometry based on shallow BP neural networks. As shown in Figure 19a, the camera image coordinates and the corresponding projector image horizontal coordinates are used as network inputs to predict the three-dimensional information of the measured object. To obtain training data, the dot calibration plate is fixed on a high-precision displacement table and stripe images of the calibration plate are captured at different depth positions. By extracting the sub-pixel centers of the calibration plate dots, and using the absolute phase, the matching points of the camera and projector images corresponding to each marker center are calculated. Figures 19c and 19d show the error distribution of the three-dimensional surface shape results of the stepped standard parts obtained by the traditional phase height conversion method < unk > < unk > and the neural networks method. The results show that the neural networks-based method can learn more accurate phase height models from a large amount of data.
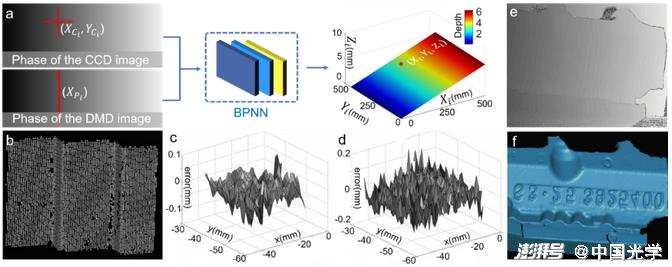
Fig. 19 a) Learning-based phase-depth mapping method: camera image coordinates and the horizontal coordinates of the corresponding projector image are used as network inputs to predict the three-dimensional information of the measured object b) The three-dimensional results of the step-shaped standard obtained by the learning-based method c, d) Error distribution of the three-dimensional surface shape results of the step-shaped standard obtained by the traditional phase height conversion method ?? and neural networks method e, f) Input phase images and output three-dimensional information of complex workpieces
Challenges and opportunities of deep learning in optical metrology
At present, deep learning has gradually "penetrated" into the discipline of computational imaging and optical measurement, and has shown amazing performance and strong application potential in fringe analysis, phase recovery, phase unfolding, etc. However, deep learning still faces many challenges in the field of optical metrology:
(1) As a data-driven technology, the performance of deep learning network output largely depends on a large number of labeled training data. The data collection process of most optical metrology experiments is complicated and time-consuming, and often the ideal true value cannot be obtained accurately and reliably after data collection [Figure 20].
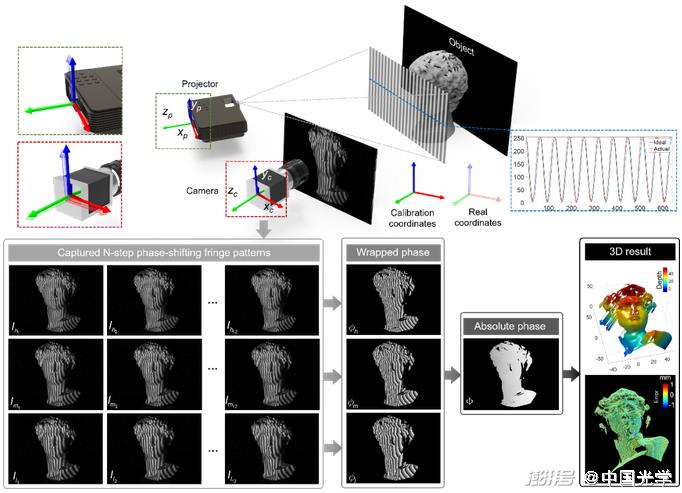
Fig. 20 The challenge of deep learning in optical metrology – the high cost of acquiring and labeling training data. Taking fringe projection profilometry as an example, the multi-frequency time phase unwrapping method is used to obtain high-quality training data at the cost of projecting a large number of fringe images. However, in practice, hardware errors, ambient light interference, calibration errors and other factors make it difficult to obtain the ideal true value through traditional algorithms
(2) So far, there is still no theory that clearly explains what structure of neural networks is most suitable for specific imaging needs [Figure 21]?
(3) The success of deep learning usually depends on the "common" features learned and extracted from the training examples as prior information. Therefore, when artificial neural networks are faced with "rare examples", it is very easy to give a wrong prediction without realizing it.
(4) Unlike the traditional "transparent" deduction process based on physical model methods, most current deep learning-based decision-making processes are generally regarded as "black boxes" driven by training data. In optical metrology, interpretability is often crucial, as it ensures traceability of errors.
(5) Since information is not "created out of nothing", the results obtained by deep learning cannot always be accurate and reliable. This is often fatal for many application fields of optical measurement, such as reverse engineering, automatic control, defect detection, etc. In these cases, the accuracy, reliability, repeatability and traceability of the measurement results are the primary considerations.
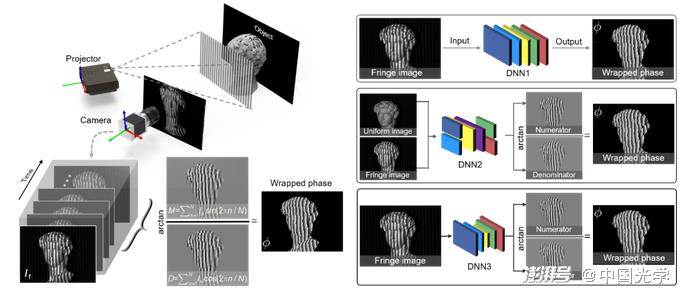
Figure 21 The challenge of deep learning in optical metrology – empiricism in model design and algorithm selection. Taking phase extraction in fringe projection profilometry as an example, the same task can be achieved by different neural networks models with different strategies: The fringe image can be directly mapped to the corresponding phase map via DNN1; The numerator and denominator terms of the tangent function used to calculate the phase information can also be output from the fringe image and the corresponding background image via DNN2; The numerator and denominator can be predicted directly from the fringe image using a more powerful DNN
Although the above challenges have not been fully addressed, with the further development of computer science and artificial intelligence technology, it can be expected that deep learning will play an increasingly prominent role in optical metrology in the future through the following three aspects:
(1) The application of emerging technologies (such as adversarial learning, transfer learning, automated machine learning, etc.) to the field of optical metrology can promote the wide acceptance and recognition of deep learning in the field of optical metrology.
(2) Combining Bayesian statistics with deep neural networks to estimate and quantify the uncertainty of the estimate results, based on which it is possible to evaluate when neural networks produce unreliable predictions. This gives researchers another possible choice between "blind trust" and "blanket negation", namely "selective" adoption.
(3) The synergy between prior knowledge of image generation and physical models and data-driven models learned from experimental data can bring more expertise in optical metrology into deep learning frameworks, providing more efficient and "physically sound" solutions to specific optical metrology problems [Figure 22].
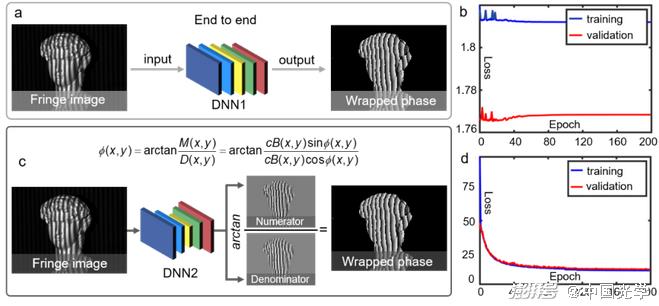
Figure 22 Introducing a physical model into deep learning can provide a more "reasonable" solution to a specific optical metrology problem. A) Directly predict the wrapped phase from the fringe image based on the end-to-end network structure (DNN1) b) It is difficult for the end-to-end strategy to accurately reproduce the 2π phase truncation, resulting in the loss function of the network not converging during training c) Incorporate the physical model of the traditional phase shift method into deep learning to predict the molecular and denominator terms of the tangent function used to calculate the phase information from the fringe image 39 d) The loss function of the deep learning network combined with the physical model can be stably converged during training
Summary and Outlook
There is no doubt that deep learning technology offers powerful and promising new solutions to many challenging problems in the field of optical metrology, and promotes the transformation of optical metrology from "physics and knowledge-based modeling" to "data-driven learning" paradigm. A large number of published literature results show that methods based on deep learning for specific problems can provide better performance than traditional knowledge-based or physical model methods, especially for many optical metrology tasks where physical models are complex and the amount of information available is limited.
But it has to be admitted that deep learning technology is still in the early stage of development in the field of optical measurement. A considerable number of researchers in this field are rigorous and rational. They are skeptical of the "black box" deep learning solutions that lack explainability at this stage, and are hesitant to see their applications in industrial testing and biomedicine. Should we accept deep learning as our "killer" solution to the problem, or reject such a "black box" solution? This is a highly controversial issue in the current optical metrology community.
From a positive perspective, the emergence of deep learning has brought new "vitality" to the "traditional" field of optical metrology. Its "comprehensive penetration" in the field of optical metrology also shows us the possibility of artificial intelligence technology bringing huge changes to the field of optical metrology. On the contrary, we should not overestimate the power of deep learning and regard it as a "master key" to solve every challenge encountered in the future development of optical metrology. In practice, we should rationally evaluate whether the large amount of data resources, computing resources, and time costs required to use deep learning for specific tasks are worth it. Especially for many applications that are not so "rigorous", when traditional physical model-based and "active policy" techniques can achieve better results with lower complexity and higher interpretability, we have the courage to say "no" to deep learning!
Will deep learning take over the role of traditional technology in optical metrology and play a disruptive role in the next few years? Obviously, no one can predict the future, but we can participate in it. Whether you are a "veteran" in the field of optical metrology who loves traditional technology, or a "newbie" who has not been involved in the field for a long time, we encourage you to take this "ride" – go and try deep learning boldly! Because it is really simple and often works!
Note: This article comes with a deep learning sample program for single-frame fringe analysis (Supplemental Material File #1) and its detailed step guide (Supplementary Information) to facilitate readers’ learning and understanding. For more details related to this article, please click https://www.nature.com/articles/s41377-022-00714-x Come and read the body of the 54-page paper.
Paper information
Zuo, C., Qian, J., Feng, S. et al. Deep learning in optical metrology: a review. Light Sci Appl 11, 39 (2022).
https://doi.org/10.1038/s41377-022-00714-x
The first author of this article is Professor Zuo Chao of Nanjing University of Science and Technology, and PhD student Qian Jiaming of Nanjing University of Science and Technology is co-author. Co-authors include Associate Professor Feng Shijie of Nanjing University of Science and Technology, PhD student Yin Wei of Nanjing University of Science and Technology, PhD student Li Yixuan of Nanjing University of Science and Technology, PhD student Fan Pengfei of Queen Mary University of London, UK, Associate Professor Han Jing of Nanjing University of Science and Technology, Professor Qian Kemao of Nanyang University of Technology in Singapore, and Professor Chen Qian of Nanjing University of Science and Technology.
references
1. G?svik, K.J. Optical metrology. (John Wiley & Sons, 2003).
2. Creath, K. V phase-measurement interferometry techniques. In Progress in optics vol. 26 349 – 393 (Elsevier, 1988).
3. Aben, H. & Guillemet, C. Integrated photoelasticity in Photoelasticity of Glass 86 – 101 (Springer, 1993).
4. Gabor, D. A new microscopic principle. Nature 161, 777 – 778 (1948).
5. Schnars, U., Falldorf, C., Watson, J. & Jüptner, W. Digital holography in Digital Holography and Wavefront Sensing 39 – 68 (Springer, 2015).
6. Gorthi, S. S. & Rastogi, P. Fringe projection techniques: whence we are? Opts. Lasers Eng. 48, 133 – 140 (2010).
7. Pan, B., Qian, K., Xie, H. & Asundi, A. Two-dimensional digital image correlation for in-plane displacement and strain measurement: a review. Meas. Sci. Technol. 20, 062001 (2009).
8. Marr, D. & Poggio, T. A computational theory of human stereo vision. Proc. R. Soc. Lond. B Biol. Sci. 204, 301 – 328 (1979).
9. Pitas, I. Digital image processing algorithms and applications. (John Wiley & Sons, 2000).
10. Trusiak, M., Patorski, K. & Wielgi, M. Adaptive enhancement of optical fringe patterns by selective reconstruction using FABEMD algorithm and Hilbert spiral transform. Opts. Express 20, 23463 – 23479 (2012).
11. Awatsuji, Y. et al. Single-shot phase-shifting color digital holography. In LEOS 2007-IEEE Lasers and Electro-Optics Society Annual Meeting Conference Proceedings 84 – 85 (IEEE, 2007).
12. Fusiello, A., Trucco, E. & Verri, A. A compact algorithm for rectification of stereo pairs. Mach. Vis. Appl. 12, 16 ? 22 (2000).
13. Zuo, C. et al. Phase shifting algorithms for fringe projection profilometry: A review. Opts. Lasers Eng. 109, 23 – 59 (2018).
14. Zuo, C., Huang, L., Zhang, M., Chen, Q. & Asundi, A. Temporal phase unwrapping algorithms for fringe projection profilometry: A comparative review. Opt. Lasers Eng. 85, 84 -103 (2016).
15. Konolige, K. Small vision systems: Hardware and implementation. in Robotics research 203 – 212 (Springer, 1998).
16. Hong, C.K., Ryu, H.S. & Lim, H.C. Least-squares fittings of the phase map obtained in phase-shifting electronic speckle pattern interferometry. Opt. Lett. 20, 931 -933 (1995).
17. Zuo, C., Chen, Q., Qu, W. & Asundi, A. Phase aberration compensation in digital holographic microscopy based on principal component analysis. Opt. Lett. 38, 1724 – 1726 (2013).
18. Langehanenberg, P., Kemper, B., Dirksen, D. & Von Bally, G. Autofocusing in digital holographic phase contrast microscopy on pure phase objects for live cell imaging. Appl. Opt. 47, D176 – D182 (2008).
19.Wang, Y. & Zhang, S. Optimal fringe angle selection for digital fringe projection technique. Appl. Opt. 52, 7094 – 7098 (2013).
20. McCulloch, W. S. & Pitts, W. A logical calculus of the ideas imperceptible in nervous activity. Bull. Math. Biophys. 5, 115 – 133 (1943).
21. Rosenblatt, F. The perceptron: a probabilistic model for information storage and organization in the brain. Psychol. Rev. 65, 386 (1958).
22. Rumelhart, D.E., Hinton, G.E. & Williams, R.J. Learning representations by back-propagating errors. nature 323, 533 – 536 (1986).
23. LeCun, Y. et al. Backpropagation applied to handwritten zip code recognition. Neural Comput. 1, 541 – 551 (1989).
24. Hinton, G.E., Osindero, S. & Teh, Y.-W. A fast learning algorithm for deep belief nets. Neural Comput. 18, 1527 – 1554 (2006).
25. Krizhevsky, A., Sutskever, I. & Hinton, G. E. ImageNet classification with deep convolutional neural networks. Commun. ACM 60, 84 -90 (2017).
26. Nair, V. & Hinton, G.E. Rectified linear units improve restricted boltzmann machines.in ICML (2010).
27. Hinton, G.E., Srivastava, N., Krizhevsky, A., Sutskever, I. & Salakhutdinov, R.R. Improving neural networks by preventing co-adaptation of feature detectors. ArXiv Prepr. ArXiv12070580 (2012).
28. Chen, J.X. The evolution of computing: AlphaGo. Comput. Sci. Eng. 18, 4 ? 7 (2016).
29. LeCun, Y., Bengio, Y. & Hinton, G. Deep learning. Nature 521, 436 – 444 (2015).
30. Ouyang, W. et al. DeepID-Net: Object detection with deformable parts based convolutional neural networks. IEEE Trans. Pattern Anal. Mach. Intell. 39, 1320 – 1334 (2016).
31. Doulamis, N. & Voulodimos, A. FAST-MDL: Fast Adaptive Supervised Training of multi-layered deep learning models for consistent object tracking and classification. in 2016 IEEE International Conference on Imaging Systems and Techniques (IST) 318-323 (IEEE, 2016).
32. Dong, C., Loy, C.C., He, K. & Tang, X. Image super-resolution using deep convolutional networks. IEEE Trans. Pattern Anal. Mach. Intell. 38, 295 – 307 (2015).
33. Long, J., Shelhamer, E. & Darrell, T. Fully convolutional networks for semantic segmentation. In Proceedings of the IEEE conference on computer vision and pattern recognition 3431 – 3440 (2015).
34. Ronneberger, O., Fischer, P. & Brox, T. U-net: Convolutional networks for biomedical image segmentation. In International Conference on Medical image computing and computer-assisted intervention 234 – 241 (Springer, 2015).
35. Yan, K. et al. Fringe pattern denoising based on deep learning. Op. Commun. 437, 148 – 152 (2019).
36. Shi, J., Zhu, X., Wang, H., Song, L. & Guo, Q. Label enhanced and patch based deep learning for phase retrieval from single frame fringe pattern in fringe projection 3D measurement. Opt. Express 27, 28929 (2019).
37. Kemao, Q. Windowed Fourier transform for fringe pattern analysis. Appl. Opt. 43, 2695 – 2702 (2004).
38. Takeda, M., Ina, H. & Kobayashi, S. Fourier-transform method of fringe-pattern analysis for computer-based topography and interferometry. JosA 72, 156 – 160 (1982).
39. Feng, S. et al. Fringe pattern analysis using deep learning. Adv. Photonics 1, 025001 (2019).
40. Feng, S., Zuo, C., Yin, W., Gu, G. & Chen, Q. Micro deep learning profilometry for high-speed 3D surface imaging. Opts. Lasers Eng. 121, 416 – 427 (2019).
41. Qian, J. et al. Deep-learning-enabled geometric constraints and phase unwrapping for single-shot absolute 3D shape measurement. APL Photonics 5, 046105 (2020).
42. Tao, T. et al. Real-time 3-D shape measurement with composite phase-shifting fringes and multi-view systems. Opt. Express 24, 20253 (2016).
43. An, Y., Hyun, J.-S. & Zhang, S. Pixel-wise absolute phase unwrapping using geometric constraints of structured light systems. Opt. Express 24, 18445 – 18459 (2016).
44. Tao, T. et al. High-speed real-time 3D shape measurement based on adaptive depth constraints. Opt. Express 26, 22440 (2018).
45. Z’bontar, J. & LeCun, Y. Stereo matching by training a convolutional neural network to compare image patches. 32.
46. Mei, X. et al. On building an accurate stereo matching system on graphics hardware. In 2011 IEEE International Conference on Computer Vision Workshops (ICCV Workshops) 467 – 474 (IEEE, 2011).
47. Pang, J., Sun, W., Ren, J. SJ., Yang, C. & Yan, Q. Cascade Residual Learning: A Two-Stage Convolutional Neural Network for Stereo Matching. in 2017 IEEE International Conference on Computer Vision Workshops (ICCVW) 878 – 886 (IEEE, 2017). do i: 10.1109/ICCVW.2017.108.
48. Montresor, S., Tahon, M., Laurent, A. & Picart, P. Computational de-noising based on deep learning for phase data in digital holographic interferometry. APL Photonics 5, 030802 (2020).
49.Li, Z., Shi, Y., Wang, C., Qin, D. & Huang, K. Complex object 3D measurement based on phase-shifting and a neural network. Opt. Commun. 282, 2699 – 2706 (2009).
50.Li, Z., Shi, Y., Wang, C. & Wang, Y. Accurate calibration method for a structured lighting system. Opt. Eng. 47, 053604 (2008).
51. Feng, S., Zuo, C., Hu, Y., Li, Y. & Chen, Q. Deep-learning-based fringe-pattern analysis with uncertainty estimation. Optica 8, 1507 – 1510 (2021).
Article reprint/business cooperation/research group submission, WeChat:447882024
Let’s read one article a day!Join >Light reading club